Note
Click here to download the full example code
Linear and Monotonic dimensions¶
In the following example, we illustrate how one can covert a Numpy array into a CSDM object. Start by importing the Numpy and csdmpy libraries.
import matplotlib.pyplot as plt
import numpy as np
import csdmpy as cp
Let’s generate a 2D NumPy array of random numbers as our dataset.
data = np.random.rand(8192).reshape(32, 256)
To convert this array into a csdm object, use the as_csdm()
method,
data_csdm = cp.as_csdm(data)
print(data_csdm.dimensions)
Out:
[LinearDimension(count=256, increment=1.0),
LinearDimension(count=32, increment=1.0)]
This generates a 2D{1} dataset, that is, a two-dimensional dataset with a single one-component dependent variable. The two dimensions are, by default, set as the LinearDimensions of the unit interval.
You may set the proper dimensions by generating the appropriate Dimension
objects and replacing the default dimensions in the data_csdm
object.
d0 = cp.LinearDimension(
count=256, increment="15.23 µs", coordinates_offset="-1.95 ms", label="t1"
)
Here, d0
is a LinearDimension with 256 points and 15.23 µs increment. You
may similarly set the second dimension as a LinearDimension, however, in this
example, let’s set it as a MonotonicDimension.
array = 10 ** (np.arange(32) / 8)
d1 = cp.as_dimension(array, unit="µs", label="t2")
The variable array
is a NumPy array that is uniformly sampled on a log
scale. To convert this array into a Dimension object, we use the
as_dimension()
method.
Now, replace the dimension objects in data_csdm
with the new ones.
data_csdm.dimensions[0] = d0
data_csdm.dimensions[1] = d1
print(data_csdm.dimensions)
Out:
[LinearDimension(count=256, increment=15.23 µs, coordinates_offset=-1.95 ms, quantity_name=time, label=t1, reciprocal={'quantity_name': 'frequency'}),
MonotonicDimension(coordinates=[1.00000000e+00 1.33352143e+00 1.77827941e+00 2.37137371e+00
3.16227766e+00 4.21696503e+00 5.62341325e+00 7.49894209e+00
1.00000000e+01 1.33352143e+01 1.77827941e+01 2.37137371e+01
3.16227766e+01 4.21696503e+01 5.62341325e+01 7.49894209e+01
1.00000000e+02 1.33352143e+02 1.77827941e+02 2.37137371e+02
3.16227766e+02 4.21696503e+02 5.62341325e+02 7.49894209e+02
1.00000000e+03 1.33352143e+03 1.77827941e+03 2.37137371e+03
3.16227766e+03 4.21696503e+03 5.62341325e+03 7.49894209e+03] us, quantity_name=time, label=t2, reciprocal={'quantity_name': 'frequency'})]
Plot of the dataset.
plt.figure(figsize=(5, 3.5))
cp.plot(data_csdm)
plt.tight_layout()
plt.show()
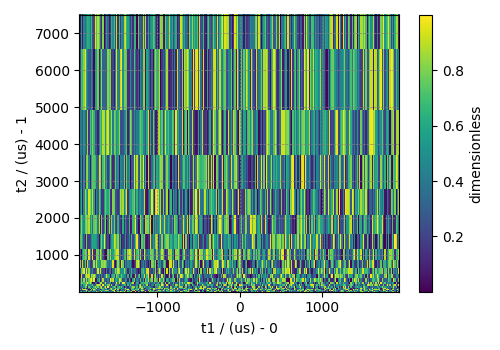
To serialize the file, use the save method.
data_csdm.save("filename.csdf")
Total running time of the script: ( 0 minutes 0.207 seconds)